Contributed by Dan Bolnick
On April 30 2025 (the same day NSF froze all funding actions), about 20 colleagues and I were crisscrossing Capitol Hill in Washington DC meeting with staff of our Senators and Representatives (and occasionally the congressfolk themselves). I was there with a delegation from the American Institute of Biological Sciences (AIBS) to ask Congress to support science in the US through continued funding at or above recent levels. Allocating funding, after all, is where Congress’ primary power lies. But in addition to our “ask”, we also came to our meetings with an “offer”. We would ask the staff, “what can we do to help the Representative or Senator push through science-friendly policy?” That offer of help changed the nature of our meetings – the aides began giving us specific advice, questions, and requests. Here, I want to convey some of the lessons I learned from those conversations. I’ll give the short social-media post ‘executive summary’ version, then elaborate below in a longer post.
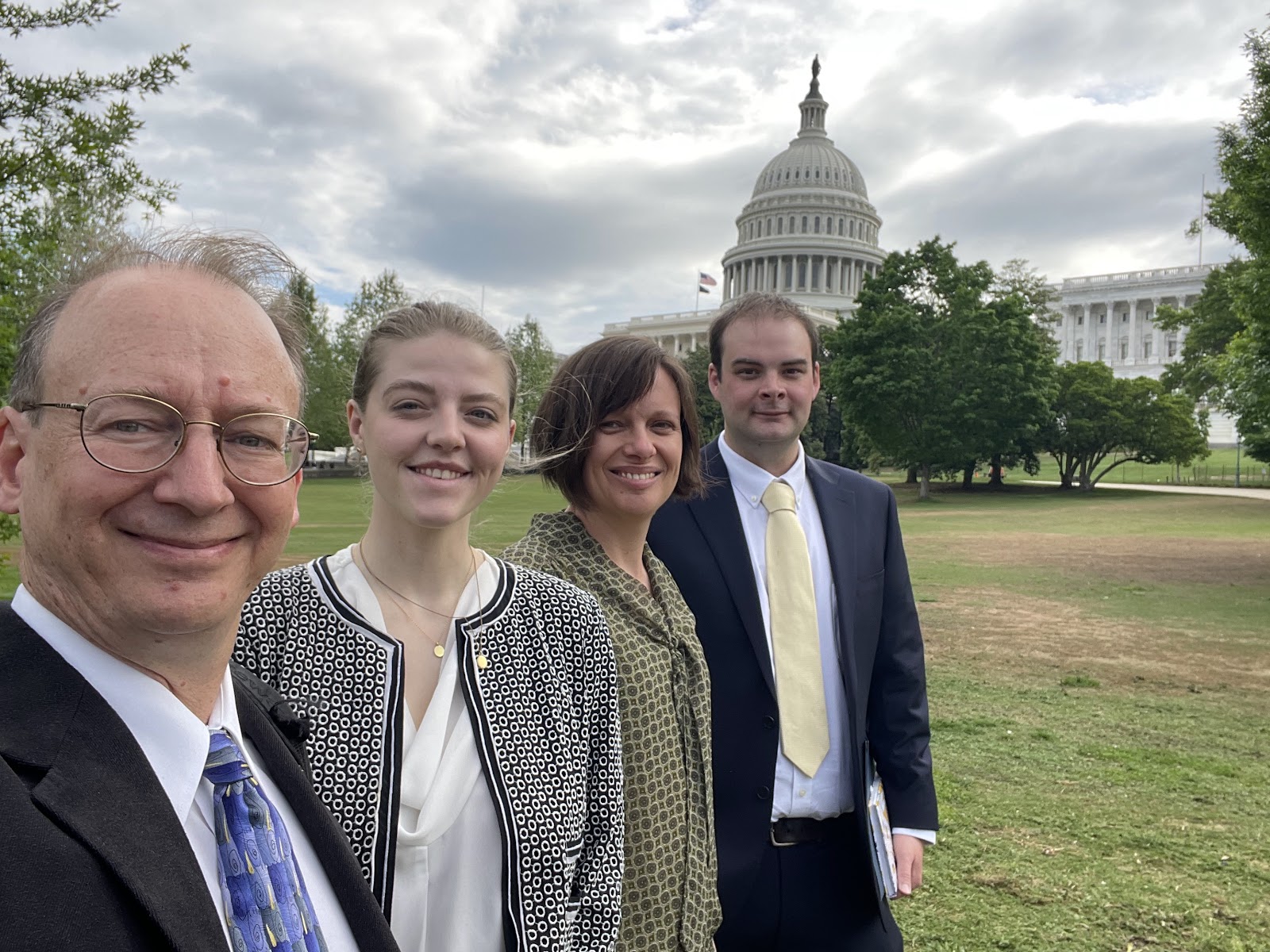
Quick version:
When asked about what we scientists can do to help federal funding of science, Congressional aides suggested the following:
1) Send your representatives specific, personal, heart-warming stories of the benefits of science funding, and also examples of the harm done by cuts to science. Be sure it connects with everyday lives in ways anyone can relate to.
2) Engage in science communication and public outreach to get the wider public excited about science, and concerned about cuts.
3) Focus your letter writing and phone calling wisely, on those in power (GOP), and on those who need to be persuaded (GOP). So those of you in “red states” are most important in advocating for science. Find common ground with opponents of science funding to try to persuade them.
4) Your reps (especially democrats) are cut off from information coming from federal agencies under the executive branch. They are learning about science cuts from us, or from the media, so when you learn about breaking examples of terminated grants, interference in free speech, etc, call or mail your reps to inform them.
5) You can ghost write material (Dear Colleague Letters, Oversight Letters, questions for committees and hearings) that your representative might find useful, and their staff are not qualified to write.
6) There is hope: there remains bipartisan support for science in Congress, they just need the spine to stand up for their beliefs. Hearing from constituents who support science, and hearing the benefits of NSF and harms from cutting it, helps strengthen their resolve.
Now, the long version…
First, a brief explanation about the AIBS Congressional Visits Day. The AIBS offers a two day training event in Washington DC each year, which I very highly recommend. This event covers some basics of science communication, the federal budget process, and how to develop a ‘script’ for meetings with congressfolk or their aides to succinctly convey your “ask”. I found the training itself to be very useful, a nice mix of presentations about do’s and don’t’s, coupled with active group work, presentation, and giving feedback on each others’ scripts. The event was attended by a couple dozen scientists – mostly graduate students and postdocs – with a strong field and organismal biology representation. At the end of the training, we split up into delegations by geographic region and had a full day of meetings with our representatives. Congressional offices tend to only book meetings with their own constituents, so my group (myself from Connecticut, plus two Massachusetts and one New Hampshire residents) met with Senators’ staff from our three states, and staff from our particular House districts. Other AIBS attendees from elsewhere in the country met with staff from southern, east coast, Midwest, southwest, mountain, and west coast representatives.
As a member of the New England delegation, we had it rather easy: every one of our Senators and Representatives had previously voted for the CHIPS and Science act (e.g., funding NSF at nearly $12 billion dollars). New Hampshire is very much a swing state where we thought it was important to clearly convey how important science funding is. There, we went with our script, which had a central “ask” (please protect science in the US) with three pillars to it (funding, protecting free speech and inquiry, and protecting the people who do science).
But for the most part, I felt a bit guilty taking 20 minutes of a busy congressional aide’s time to argue for something they were likely to do anyway. So we pivoted to giving more time to the “offer”. And that’s where things began to get interesting. Here are some of the responses that we got, when we asked what we as scientists (and you, too) can do to help congress help science:

1. Tell the reps stories.
This was the most universal theme. “Stories, stories, stories,” said one aide. Ultimately, lawmaking and funding are about how constituents feel, and making their constituents feel heard and supported. And a key rule in politics is that emotionally resonant stories are far more effective at winning hearts and minds, than numbers can be. So, call or write to your congressperson with stories about how science funding is benefitting their constituents. This gives your representative a supply of stories that they can draw on in conversations with skeptical colleagues, on speeches, in press conferences.
The stories need to be something that they, and a random constituent, would value. If I just say that the money makes it possible for me to study fish speciation, that’s not going to strike a non-biologist as valuable. So, what kinds of stories connect for a politician? Stories that relate to their constituents’ jobs, workforce training, income, health, safety, and quality of life. To some extent, that means applied research producing innovations that help small businesses or agriculture or fisheries, conservation, health care advances, technology, patents. Applications. Now, many of us don’t actually pursue applied research (I sure don’t). But “basic” science (or what I prefer to call discovery science) is nevertheless a great engine for producing unexpected benefits. You might not have any applications from your work – yet. But might you in the future? I’ll give a couple examples of stories that I prepared for my congressional meetings, just for a flavor, at the end of this post.
Even if you aren’t doing applied work you can tell personal stories about employment. I told my House Representative’s aide that I had brought in $X in research funding, that we largely spent employing students and research technicians, generating over 250 jobs in my district over the years. Those employees spent their income within the Representative’s district. And when they did research they often bought supplies from local businesses. So those research dollars coming into the Representative’s district are spent several times over, contributing to the local economy.
Workforce training is important to representatives also. Academics sometimes complain that we train more PhDs than there are faculty jobs to receive them. But that’s a strength, not a weakness – those ‘excess’ PhDs may be the most important thing we do, because they go on and use their skills in data analysis and communication to contribute to the US economy. As an example, I brought a brilliant student into the US from another country, and after she graduated with a PhD in biology she used her statistical savvy to work as a data scientist, first at AirBnB, then Oculus, and now as a leading data scientist at Meta, contributing to the growth of our tech economy. Then, there are all the undergraduates who we work with in the classroom, lab, and field, who go on to use the skills we teach them in do work as doctors, pharmacists, epidemiologists, start-up company owners, K-12 teachers, and far more. Stories of these former students’ later careers convey the key role NSF funding has in creating an educated workforce needed for the modern economy.
That said, statistics do help sometimes. An aide for one senator with a strong foreign-affairs focus clearly took notes when I gave her specific numbers on how US spending on science R&D compared to Chinese spending on R&D, and the opposing trends (US cutting R&D, China increasing it dramatically). Another took notes when I mentioned specific numbers about grant funding received and number of people employed. Still others liked a colleague’s numbers about the seafood trade deficit ($20 billion, twice the total spending on NSF). I find this report on the economic value of federal R&D funding especially compelling: https://aura.american.edu/articles/report/Preliminary_Estimates_of_the_Macroeconomic_Costs_of_Cutting_Federal_Funding_for_Scientific_Research/28746446?file=53480237 from the Institute from Macroeconomic & Policy Analysis.
2. Engage in public outreach and science communication.
Congressional support of science funding will pretty much always be as strong, or as tepid, as public support for science. Many of us have struggled, at one time or another, to explain to our relatives (or, friends) what we do, and why it is interesting. If your own relatives don’t understand your work’s value, they can at least appreciate that it is giving you some income (I hope). But now imagine a random tax payer who is equally skeptical of the value of your work, but actively dislikes the idea of their tax dollars going to your salary. We need that random person to see the value in your science, so that they are motivated enough to write to their own representatives in support of science funding. Or, at least, not object to science funding. To bring that random person on board, we need effective science communication aimed at the public. Often that might take the form of articles or OpEds in local papers, local news channels, which reach the constituents in your own district. Convince people that grants aren’t wasteful, that the money is going to something that generates something they value. You might value knowledge for its own sake, but don’t assume others do, so think about value also in terms of economic growth, jobs, health, safety, conservation. One congressional aide emphasized these “tangible impacts” multiple times, connecting science to benefits that non-scientists will feel in their “everyday lives”.

3. Focus advocacy efforts wisely.
One of the things that surprised me is how focused Congressfolk are, on their own constituents. If you want to schedule a meeting with them, or their staff, you must live in their district. It’s not enough to have information relevant to their district. Nor is it enough to do research or spend funds in their district. Therefore, our New England delegation met with exclusively Democratic Senators and Representatives (we didn’t have anyone from Maine along). So we were preaching to the choir (a phrase that at least two aides used, which felt a tiny bit like a rebuke for wasting their time). But those same aides emphasized that what we really should be doing is pushing our peers in swing states and Republican-represented states to write letters, call, and arrange similar meetings. Focus our advocacy efforts on the people currently in power, who chair committees and have a majority of votes. The Democrats we met with seemed to feel nearly as helpless as we do. Also focus advocacy on the anti-science representatives who need to hear from constituents who disagree with them. So, we really need those of you who are in Republican-controlled districts to do some of the heavy lifting here. This isn’t just folks with Republican senators or house members; you may have a Democratic Congressional representative but a Republican governor (looking at you, New Hampshire) who can be pressured to express concerns to his party members in Washington DC. The rest of us can help out by working with our colleagues to hone a message, help you practice your delivery. But, it is crucial that our red-state colleagues be especially vocal. And given the current climate of fear of reprisals, we really need to encourage our senior colleagues who are retired, or nearing retirement, to lead the charge here. They don’t have to fear having a grant cancelled, or their employer targeted, so have far more freedom to speak their minds.
When reaching out to a more skeptical audience, there are some things to keep in mind. First, tell stories that have emotional and economic resonance (see point 1, above). Second, find common ground. There’s always something that your opposite will value, that you can draw on. One member of our delegation works on population genetics of marine organisms, and could point to the seafood trade deficit: we spend 20 billion dollars more on buying foreign seafood, than we export. That’s twice the entire NSF budget! So if we can spend a bit of NSF money to improve our seafood industry by better matching genotypes of aquaculture organisms (e.g., oysters) to warming water climates, that’s an investment that can improve economic productivity and cut into the trade deficit. Republican politicians are currently obsessed with efficiency, so we can make the point that grant terminations are downright inefficient. If you build a house, and stop just before you put the roof on because you want to stop spending money, that’s not savings, that’s wasting the funds you’ve already put in, without seeing any of the benefit that would later accrue. Hunters and gun lobbyists value nature. And everyone values health. If you are willing to go down a slightly jingoistic path, you can raise alarms about how US government funding for scientific research & development is declining as a share of the economy, whereas in China it increased by over 8% just last year. They are on track to spend 2.6% of their budget on R&D, compared to our 0.6%. Lots of republicans are worried about geopolitical competition from China, and a powerful argument for them is to invoke memories of Russian achievements during the Sputnik area, and how that spurred scientific funding that allowed the US to dominate in science globally. Argue that this is a new Sputnik moment. Find that common ground and exploit it to explain why science funding, and freedom of inquiry, yield benefits that your interlocutor wants.
The good news is, after our congressional visits day we reconvened with a few of our colleagues from other regions of the US, for dinner. They reported that even their Republican representatives were broadly supportive of continuing NSF, NIH, and NOAA funding. During the last Trump administration, he proposed a 50% cut to NSF, but congress ignored that request and passed a budget that gave NSF a slight increase.
4. Pass along breaking information
I was astonished to hear that federal agency staff have been largely prohibited from communicating with Democratic members of Congress. This means that our Democratic representatives are mostly in the dark about grant terminations, prohibited speech, and other political interference within science funding agencies. They only know what they learn through the news (which is often significantly behind on breaking events in science), and from what their constituents tell them. So please, if you have specific evidence of something that congress should know about (a terminated grant, impounded funds, grant programs being mothballed), let your representatives know about it. It may be that they hear it first from you. For example, some of the staff we talked to did not know that the NSF only awarded half the usual number of Graduate Research Fellowships – that’s over 1000 of our best students who normally would have received salaries to pursue innovative science. That’s less income for their districts, and less economic growth as a result. Other staff did not know that grant programs earmarked by congress had nevertheless been archived, an illegal impoundment of Congressionally-allocated funds. So, every grant termination, every cancelled review panel, let your representatives know! This is especially important because the US Constitution gives Congress the “power of the purse” to determine spending levels. In practice, the White House is using a strategy called “impoundment control” (which, I am told is illegal, though I am not an expert on this point) in which they simply drag their feet on actually spending allocated funds. Congress needs to know of specific examples of impoundment (and the harm it causes) if they are to pursue protecting their constitutional role in government.
5. Ghost write
Your congressional representative has a small staff of mostly 20 year olds, very few of whom have any scientific training. Those aides don’t have the expertise, nor the time, to provide their boss with detailed text that can be used to move forward legislation on science topics. But you do. We were told by an aide to Elizabeth Warren, that we are welcome to provide ghost-written documents that the Senator could use. For example, within the Senate, and within the House, there are “Dear Colleague Letters” that express a representative’s intent to support funding for a particular item in the budget, at a particular amount. For instance, there is currently a Dear Colleague Letter within the House of Representatives written by Representatives Neguse and Fitzpatrick calling for 9.9 billion in funding for NSF – that’s a shade more than the last budget, though less than the CHIPS and Science Act of 2023 ($11.96 billion), and is probably our best case scenario. These Ddear colleague letters get signed by other reps, as a way of gauging the level of support for a topic before a vote. These are an important tool for selecting subjects to legislate and fund, and building political consensus. What surprised me was that Warren’s aide said they are willing to see drafts of Dear Colleague Letters written by us, their constituents, which they might then use. In a congressional office that is thin on science expertise and short on time, this can be a powerful tool for moving forward congressional action on a topic you care about. Similarly, we constituents can ghost write Oversight Letters, which congressfolk can send to federal agencies asking for information and explanations. Finally, when there is an upcoming Congressional hearing on a topic, your Senator or Representative has a chance to ask hard questions of people like NSF or NIH directors, DOGE staff, or whoever is being grilled that day. You can submit questions that you would like your representative to ask during these hearings, of people who are testifying or questions they might pose to their fellow representatives.

These are grim times for science to be sure. US federal funding of science has been a cornerstone of nearly a century of global scientific leadership and economic growth. That leadership is quickly being squandered and once lost may not be regained (for example, Germany never recovered the global scientific leadership it had before World War 2, though now many US scientists are looking wistfully across the Atlantic). But let’s not cede the field uncontested. You have allies in Congress and in government, you simply need to give them the information and support that they need to be effective at achieving our common goals. That requires time and effort from you, but what better use of your time could there be at this juncture in history?

Example Story 1: 35% of deaths involve tissue damage called fibrosis. This is a build up of scar tissue in or around organs such as lungs, heart, or liver. I didn’t set out to study fibrosis. I began as an evolutionary biologist studying how fish split into new species. While pursuing NSF-funded research about evolution of fish in small coastal lakes, we stumbled on something unexpected: fish in some lakes experience severe fibrosis, fusing together all their organs. In other lakes, the same fish species shows no fibrosis at all, or can recover from prior fibrosis (whereas in humans the disease is irreversible). Today, my NIH-funded research team uses this same small fish to study the genetics and cellular causes of fibrosis, hoping to discover tools to prevent, or reverse, this pervasive immune disease. This is an example of how NSF funding for discovery-oriented research, driven by mere curiosity, can yield benefits that may launch new therapies and ultimately improve human well-being. The combination of federal investment in discovery-oriented research at NSF, and applied research at NIH, is a powerful combination that drives innovation for long-term economic growth in the US, and to improve health and well-being of your constituents.
Example Story 2: Funding science at NSF benefits your constituents and the nation. I’ll use my own team as an example: In 2 decades as a professor I’ve brought my university $XX million in funding, from NSF, NIH, and private foundations. That money generates new knowledge – for instance, I study evolutionary biology but this led me to research on an immune pathology contributing to millions of deaths in the US. But the funds have a much wider impact. We buy research supplies from local businesses and equipment from start-ups. My lab alone has employed over 250 people – students, postdocs, technicians whose income contribute to the local economy. And the skills they learn in the process contributes to the CT workforce: my students have become statisticians, doctors, brewers, epidemiologists, K-12 STEM teachers, and start-up owners.
Example Story 3: Let me give you an example of how NSF funding for discovery-focused science has benefitted your constituency. My student ------- moved to CT where she won a prestigious NSF graduate research fellowship to study evolutionary biology. This fellowship gave her freedom to explore different research directions, and she eventually started research on yeasts that live on apples in nearby orchards. In the process of studying how yeasts evolve to attack particular apple varieties, she unexpectedly found a poorly known species of yeast. In the lab, she bred it for cider production, creating a new commercial product we are bringing to market with LongView Cider House at Rodgers Orchards in New Britain. NSF support for her salary (which she spent living in your district) made it possible for her to help a family farm and a start-up company in your district. NSF grants and fellowships can often have this kind of unanticipated benefit, and contribute to economic growth and prosperity. A recent study by the Macroeconomic Policy Institute confirms what we have long known, that public funding of science has large economic benefits. Conversely, they found that “A 25 percent cut to public R&D spending would reduce GDP by an amount comparable to the decline in GDP during the Great Recession”. NSF is facing the possibility of 55% budget cuts. NIH faces 45% cuts. This year NSF awarded only half as many Graduate research fellowships. Postdoc fellowships are getting cut. Grants are being revoked arbitrarily. We need your support for maintaining, and growing, science funding, for the good of your constituents’ prosperity, health, quality of life, and yes, education and new knowledge.